Given the high-dimensional compositional space involved in materials design, machine learning tools based on published data that could guide the design of experiments would enable an efficient exploration of the design space and arrive at optimal formulations in the shortest possible time and with fewer experiments to perform.
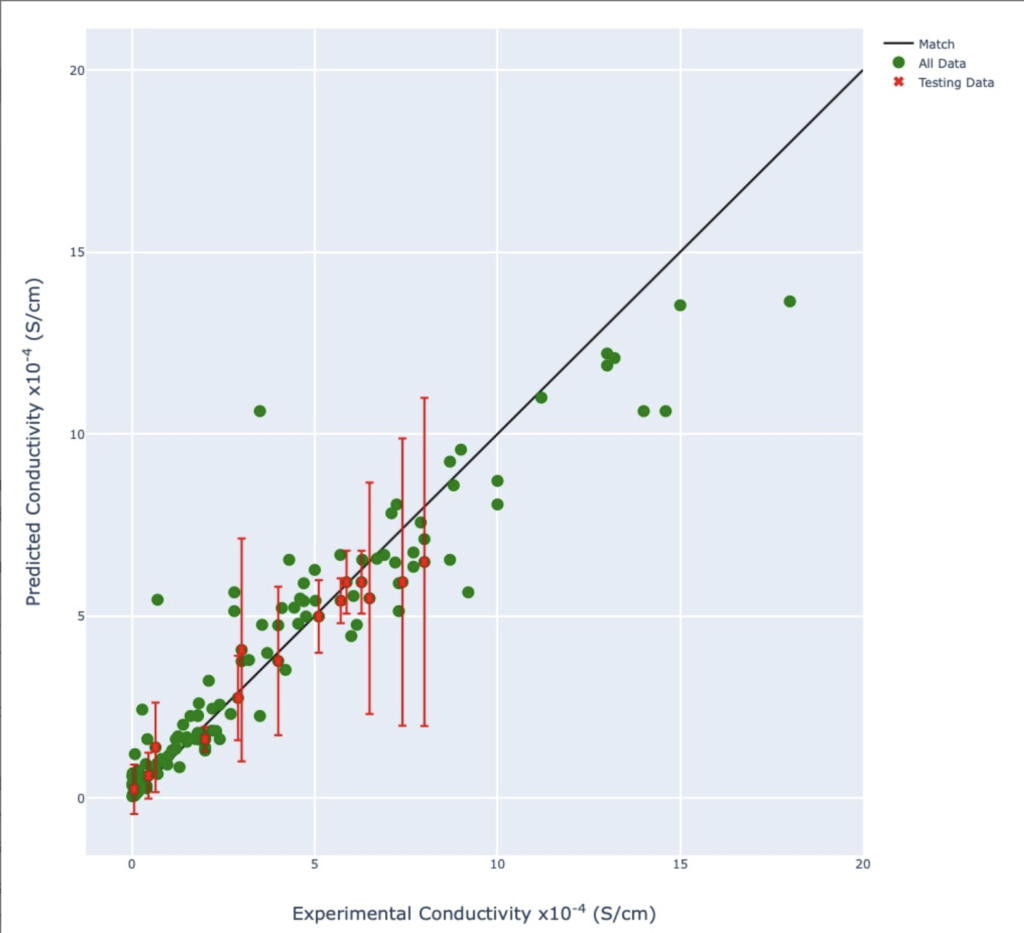
Sequential Learning for Materials Design
The Jupyter Notebooks in this tool implement methods developed by Citrine Informatics for materials design. Users can modify the notebooks to explore different models, try new ideas and adapt them for their own problems.
The examples under Sequential Learning seek to solve materials design problems (posed as a maximization or minimization problem) with the fewest number of experiments possible. In the examples below, we start with a given number of previously performed experiments, a random subset of which is revealed at the beginning of the exercise. The goal is the find the optimal experiment in the smallest possible number of trials
Website created by The Strachan Group. Powered by WordPress. 2020